inductive proximity
- time:2024-10-14 14:24:44
- Click:0
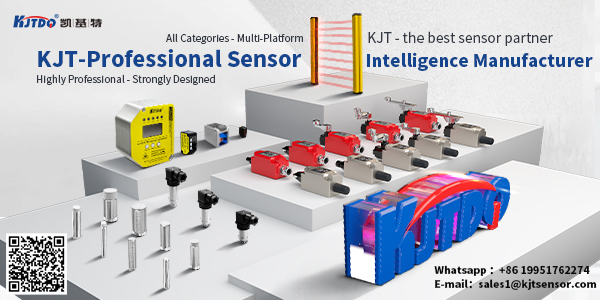
Inductive Proximity: A New Frontier in Data Analysis
In the ever-evolving world of data analysis, new techniques and methodologies are constantly emerging. One such technique that has been gaining attention in recent years is inductive proximity. This innovative approach to data analysis offers a unique perspective on the relationships between different variables, allowing researchers and analysts to gain valuable insights into complex datasets. In this article, we will explore the concept of inductive proximity and its potential applications in various fields.
Understanding Inductive Proximity
At its core, inductive proximity refers to the process of identifying patterns and relationships between variables in a dataset based on their spatial and temporal proximity. Unlike traditional deductive approaches, which rely on pre-existing hypotheses and models, inductive proximity allows analysts to uncover hidden structures and associations within data without prior assumptions. This enables a more flexible and adaptable approach to data analysis, as it allows for the discovery of previously unknown relationships and interactions between variables.
Applications of Inductive Proximity
The applications of inductive proximity are vast and varied, spanning across multiple industries and disciplines. Some potential use cases include:
- Social Network Analysis: By examining the spatial and temporal proximity of social media interactions, analysts can gain insights into the structure and dynamics of online communities, as well as identify influential users and potential influencers.
- Environmental Science: Inductive proximity can be used to analyze patterns in environmental data, such as air quality or water quality, by examining the spatial and temporal relationships between different pollutants and their sources. This can help identify areas with high pollution levels and inform targeted interventions to improve environmental health.
- Healthcare: In the field of healthcare, inductive proximity can be used to analyze patient data, such as electronic health records or wearable device data, to identify patterns and trends in disease progression and treatment outcomes. This can help healthcare providers make more informed decisions about patient care and develop personalized treatment plans.
- Finance: By analyzing the spatial and temporal proximity of financial transactions, inductive proximity can be used to detect fraudulent activity or identify potential investment opportunities. This can help financial institutions improve their risk management strategies and optimize their investment portfolios.
Challenges and Limitations
Despite its potential benefits, inductive proximity also presents certain challenges and limitations that must be considered. One major challenge is the need for large amounts of high-quality data in order to accurately identify patterns and relationships. Additionally, the interpretation of inductively derived insights can be subjective and dependent on the specific context and goals of the analysis. Finally, as with any data analysis technique, there is a risk of overfitting or discovering spurious correlations that may not hold up under further scrutiny.
Conclusion
In conclusion, inductive proximity represents an exciting new frontier in data analysis, offering a fresh perspective on the relationships between variables and the potential for discovering previously unknown patterns and associations. While there are certainly challenges and limitations associated with this approach, its potential applications in fields such as social network analysis, environmental science, healthcare, and finance make it a promising area for further research and development. As our ability to collect and analyze large volumes of data continues to grow, it is likely that inductive proximity will play an increasingly important role in shaping our understanding of complex systems and enabling more effective decision-making across a range of domains.